Mind the Gap: Navigating the security data network
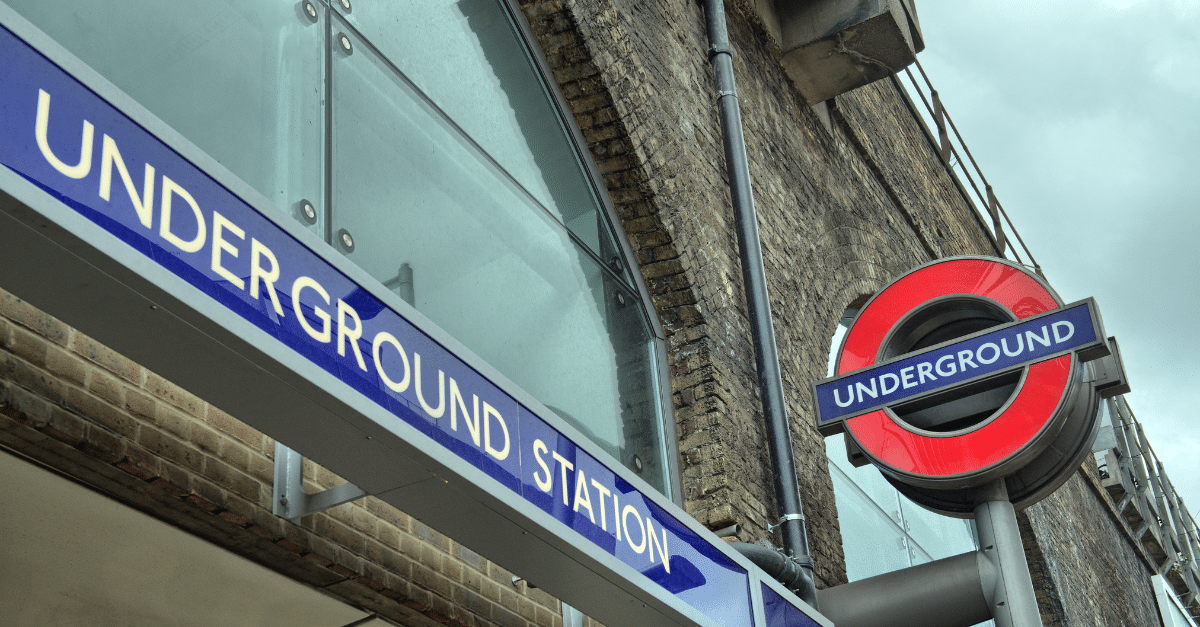
At the heart of what we do at Noetic is security data. We aggregate data from existing security and IT management tools to highlight security coverage, provide context to the incident response team, correct control drift and more. This is the fundamental role of a cyber asset attack surface management (CAASM) solution and, as such, it requires us to understand and work with different data for a wide range of cyber tooling such as EDR (Endpoint Detection & Response), vulnerability scanning, cloud security posture management (CSPM), application security and related technologies.
A common discussion I find myself having with security leaders is how we are different from other data-centric security platforms they are using today, particularly security log management platforms such as SIEM tools or security data lakes. This often stems from an understandable misconception about the roles these different systems play as part of the security architecture. The fundamental difference here is between ‘state’ data and ‘event’ data.
Tube maps and real-time travel information
When I think about how Noetic, as a data aggregator, interacts with other security data platforms, it’s useful to find a workable analogy. As a proud transatlantic company, my go-to example for this is the Transport for London (TFL) Network.
The TFL network has different services and modes depending on use case and has grown significantly through acquisition and consolidation. The most famous of the transport lines is the London Underground (Tube)— the world’s oldest underground passenger railway. Today, it is complemented by bus networks, the overground train system, trams, river services and London’s famous taxi, the black cab.
The idea here is that by having these different layers, the system builds in options, resiliency and “right tool for the right job”. This is known as ‘intermodal journey planning’.
Data aggregators are the linchpin in any large system, allowing cross silo views of the data, their relationships and context within the system. When we talk about state or event data, these two forms of data collide when you go to take a journey— the first part is the relationships.
Let’s say, for example, I want to travel from London Bridge in the city of London to Green Park near Buckingham Palace. Having a map of the “world” is useful along with the relationships between them, without this I’m likely to get lost pretty quickly.
But we also need the right context to start to plan our journey. I am probably starting off from a specific place like a restaurant near London Bridge and heading to a shop or pub near Green Park station. So, if we’re starting at those origin points, knowing what the most convenient station for me is key.
We then have the time-based aspect, maybe I need to be there in 30 minutes, this creates another set of questions, such as:
- When is the next tube?
- Are there any disruptions?
- Which is the busiest line?
- When is the last tube?
These are all event-based questions with event-based answers like:
- A train has arrived at an earlier station, so it will be at London Bridge in 2 minutes.
- There is a signaling fault, so this line is delayed.
- There is a festival around the station, so no passengers can leave the train there.
All these events are relevant, and key to the decision-making process, but they are incredibly challenging to use to build up a map of the world. They are more real time and actionable, but only provide fragments of the overall picture.
Event vs State Security Data
For cybersecurity, event data is key. It provides us with near real-time alerting of threats, remediations, issues or state changes. However, often overlooked is how bad it is at supplying insights into the overall ‘state of the world’.
Going back to the TFL analogy, if we only looked at event data and a station never had any problems, would we even know that it existed? If we only consider specific tube lines arrival times, how can we work out where we need to change tube lines? The other side of this is that state data is bad for alerting you to real-time changes.
This also relates to the relevant decision horizon. We want state data to enable longer-term decision making such as deciding which tube station is the best to live near for a commute. Inversely, for the event-oriented use case; should I leave my house now to get the less busy train? Or when is the next train arriving?
To bring this back to cybersecurity, event data is extremely poor for trying to build a picture of security posture. It will tell you that a user has repeatedly failed to login but not whether that user has MFA (Multi Factor Authentication). It’s the state data that will tell you if MFA is currently enforced.
The same is true for whether a device is currently scanned by a vulnerability management platform or has an endpoint agent installed to protect against ransomware. But event data does build a great forensic and detection picture to demonstrate that a device is emitting indicators of compromise and that the user on the device has been brute forced.
One of the reasons we founded Noetic was to better align these two models. The reason that the device has been successfully compromised could be a known, exploitable vulnerability that has remained unpatched because that host has not been scanned, so the security and IT teams were unaware of the risk.
By using state data to make ‘longer horizon’ security posture decisions and increasing security hygiene, we can reduce the blind spots in our mirrors. In pairing that with great event-based detection, we start to create real defense in depth.
These technologies are complementary in the same way the tube map and the real-time information screens are to your overall journey. You need a combination of a map of the world (state) and the flow of change data (event).
To better understand what your enterprise security tube map looks like according to the Noetic platform, register for a live demonstration or book a 1:1 meeting below.